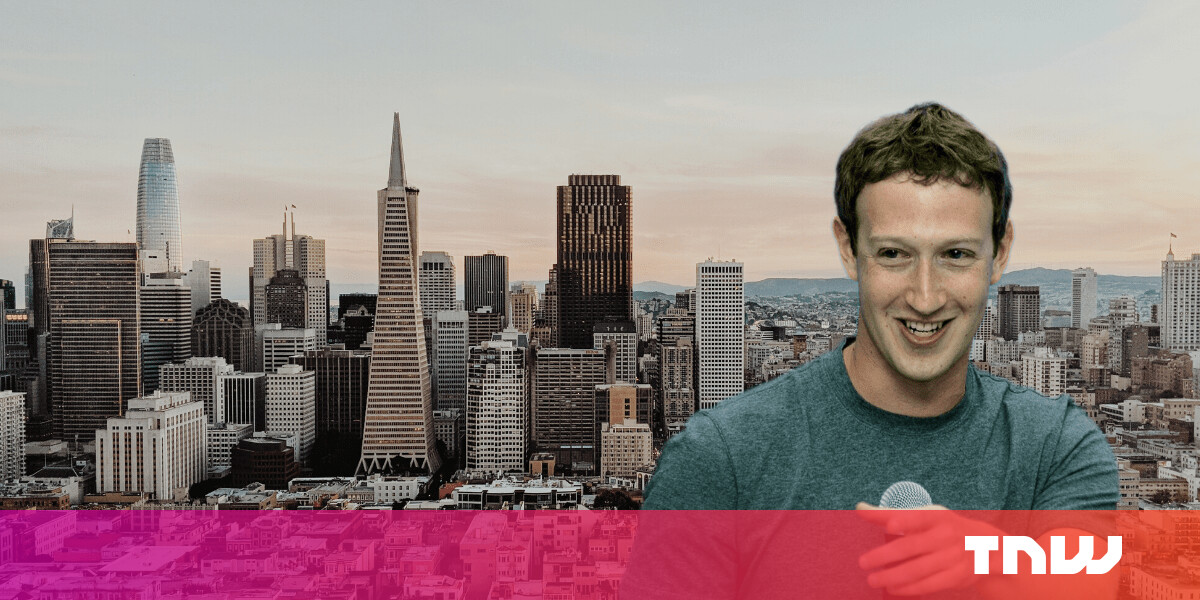
Introducing his students to the study of the human brain Jeff Lichtman, a Harvard Professor of Molecular and Cellular Biology, once asked: “If understanding everything you need to know about the brain was a mile, how far have we walked?” He received answers like ‘three-quarters of a mile’, ‘half a mile’, and ‘a quarter of a mile’.
The professor’s response? “I think about three inches.”
Last month, Lichtman’s quip made it into the pages of a new report by the Royal Society which examines the prospects for neural (or “brain-computer”) interfaces, a hot research area that has seen billions of dollars of funding plunged into it over the last few years, and not without cause. It’s projected that the worldwide market for neurotech products — defined as “the application of electronics and engineering to the human nervous system” — will reach as much as $13.3 billion by 2022.
So, despite our admitted lack of understanding, it seems the brain is a new and significant frontier for tech-pioneers looking to reinvent — and perhaps irreversibly influence — the way we interact with the world.
The Royal Society report speculates:
Mental health conditions could be treated by using interfaces to target relevant parts of the brain, bringing relief to the hundreds of millions worldwide who have depression. Even Alzheimer’s disease, which has proved resistant to conventional therapies, might be halted or reversed.
Outside of medical use:
People could undergo ‘whole brain diagnosis’ to identify their unique talents and challenges. Today’s ‘brain training’ computer games, whose impact is debated, might give way to demonstrably effective ‘brain cleaning’ or ‘mind gym’ sessions to keep minds sharp and creative.
Neural interfaces offer myriad possibilities to enhance everyday life. We could use our minds to open doors, turn on lights, play games, operate equipment or type on computers.
Then there are opportunities to enhance or supercharge the brain itself. Implants, helmets, headbands or other devices could help us remember more, learn faster, make better decisions more quickly and solve problems, free from biases…
Mood, knowledge and memory could be securely and confidentially backed up or uploaded to a digital cloud.
I know, it’s a lot. And I’ve omitted the references to telepathy, the potential merging of humans with artificial intelligence, and the option to hook your neural interface up to that of another animal, like a bird.
To a sci-fi nut, this must all sound like manna from heaven. To the rest of us, it’s likely to be a little bewildering (to say the least). So, is this a real proposition? Or just the (fairly creepy) wishlist of some over-ambitious Silicon Valley nerds?
The truth is that it’s difficult to tell what the long-term trajectory for brain-computer interfaces will be but, to a degree, they are already here. Though still fairly elementary, we currently have drones and artificial limbs that can be controlled using the brain alone, as well as headsets that boost concentration and memory. Some of these technologies are invasive, but many are not. Some record and react to brain activity, some stimulate it, and some do both.
Reassuringly, it’s non-invasive technologies that look to be headed for commercial distribution. Most of these are re-imaginings of the electroencephalogram (EEG), a system that monitors and records electrical impulses in the brain. One of the leaders in the commercial space, CTRL-Labs, specifically focuses on what it calls ‘intention capture’. Their product is a electromyogram (EMG)-based wristband, which can respond to electrical signals as they activate in a user’s arm muscle. At the moment, the company’s demo has a player controlling a simple game using only this impulse detection and no physical movement (take a look).
If you’re cynical about how far this could go, you should know that Facebook acquired CTRL-Labs last month, and just a couple of weeks ago leaked transcripts from Mark Zuckerberg’s internal meetings reinforced the firm’s keen interest in brain-computer interfaces.
Giving his thoughts on Elon Musk’s Neuralink project, Zuck says:
I am very excited about the brain-computer interfaces for non-invasive. What we hope to be able to do is just be able to pick up even a couple of bits. So you could do something like, you’re looking at something in AR, and you can click with your brain. That’s exciting… Or a dialogue comes up, and you don’t have to use your hands, you can just say yes or no. That’s a bit of input. If you get to two bits, you can start controlling a menu, right, where basically you can scroll through a menu and tap. You get to a bunch more bits, you can start typing with your brain without having to use your hands or eyes or anything like that. And I think that’s pretty exciting. So I think as part of AR and VR, we’ll end up having hand interfaces, we’ll end up having voice, and I think we’ll have a little bit of just direct brain.
If a little bit of “direct brain” doesn’t bother you, it’s worth looking ahead to the possibilities that extend beyond basic control of an elementary system.
For example, we already have neural systems that can read moods and emotions. Last year, The South China Morning Post reported that this kind of technology had been deployed by Chinese firms looking to monitor employees for signs of anger, anxiety or depression using devices built into headwear and hats. And perhaps even more impressively (or disturbingly), researchers at Kyoto University in Japan have been able to use a deep neural network to convert brain signals from an fMRI scan (used to map neural activity) into an image that contains many of the shape and color characteristics as one viewed by the subject of the scan.
This is all just to say that these types of systems are unlikely to cease development once they provide the capabilities to click or scroll in Mark Zuckerberg’s AR hellscape.
The Royal Society report makes sure to flag some early concerns. Most rational-thinking people won’t be too far behind them: What would it mean if an external company or government could gain access to our moods, or even our thoughts? How might human privacy — and indeed autonomy — be protected in if these technologies became ubiquitous? How can we ensure that they wouldn’t be weaponized by bad actors or governments to influence and control entire populations? (And is it okay if they only want to subliminally coax us to eat more healthily or respect the rules…?)
It’s not hard to think of governments that will be watching the progression of this technology very keenly.
Though it’s only fair to weigh risks against benefits before eagerly ringing the alarm bell, even here there is ambiguity. The benefits of commercializing this technology seem extremely limited, at least on the face of it. Gameplay? Fitness? Hands-free navigation of augmented or virtual reality environment? None of these feel like strong arguments for selling access to our brains.
But what about neural interfaces that could improve memory or concentration, making us super productive in life and work? Presumably, one could make the case that this is a worthwhile trade? Well, incidentally, completely separate research released just after the Royal Society report should urge caution around attempts to enhance such functions.
A new journal in Science published findings that appear to affirm the long-held theory that there is an active “forgetting mechanism” which kicks in while we sleep. The study found that when researchers suppressed neurons that produce the naturally occurring hypothalamic melanin-concentrating hormone (MCH) in mice, their memory performance actually increased. In other words, without this unnatural suppression these hormones act very deliberately to impair — or “modulate” — our memories.
This is a biological addition, not some kind of “lack” that we must compensate for with technology. We might safely assume that it serves some worthwhile evolutionary purpose.
Indeed, there is good reason to believe that if we didn’t forget we would live in a perpetual state of confusion, our brains awash with confusing superfluous information. One curious story that speaks to the chaos of the ever-remembering mind is that of the man who became known as subject S; a young Moscow-based journalist (later identified as Solomon Shereshevsky) who approached neuropsychologist Dr. Alexander Luria in 1929 with a very peculiar problem: he could not forget.
According to Luria’s reports, subject S. was able to remember foreign poems, scientific formulas, and enormously long strings of words and numbers decades after he had been told them. He recited them to perfection every time Luria tested him.
Great asset, eh? To never forget a name at a cocktail party, miss a birthday, fail a test on a fact or formula you already learned? To remember your own human life with crystal clarity rather than with the foggy haze that tends to wash over even our dearest memories?
Not so. According to the New York Times:
S.’s ability to remember was also a hindrance in everyday life. He had a hard time understanding abstract concepts or figurative language, and he was terrible at recognizing faces because he had memorized them at an exact point in time, with specific facial expressions and features. The ability to forget, scientists eventually came to realize, was just as vital as the ability to remember.
Who knows what psychological or neural confusion could eventually be brought on by using brain-computer interfaces to optimize evolutionary facets…
But we probably shouldn’t run screaming for the hills just yet. These systems are in their infancy, and there have been incredible breakthroughs in the research that should yield great benefits for people with mental and physical impairments. Nevertheless, The Royal Society are right to get ahead of the ethical and moral dilemmas that will accompany the commercialization of this type of technology. It is unfamiliar terrain, and allowing a system to intervene on our physical and mental capacities is an unprecedented encroachment that could easily turn sour. Certainly if we are to judge by the ways technological intelligence and surveillance have been wielded so far.
For now we should keep a close watching brief on how this technology develops, as well as any-and-all proposals for its use. One thing seems to be true, if we thought society had already reached its technological saturation point, we “ain’t seen nothin’ yet.”
This article was originally published on Towards Data Science by Fiona J. McEvoy, a tech-ethics researcher and founder of YouTheData.com. She examines the use of technology, A.I., and data in our society.
from The Next Web https://thenextweb.com/syndication/2020/01/02/how-silicon-valley-wants-to-fuck-with-our-brains/