What is AI? Even Elon Musk Can’t Explain
Artificial intelligence is hard to define — because the field is broad and the goals keep moving
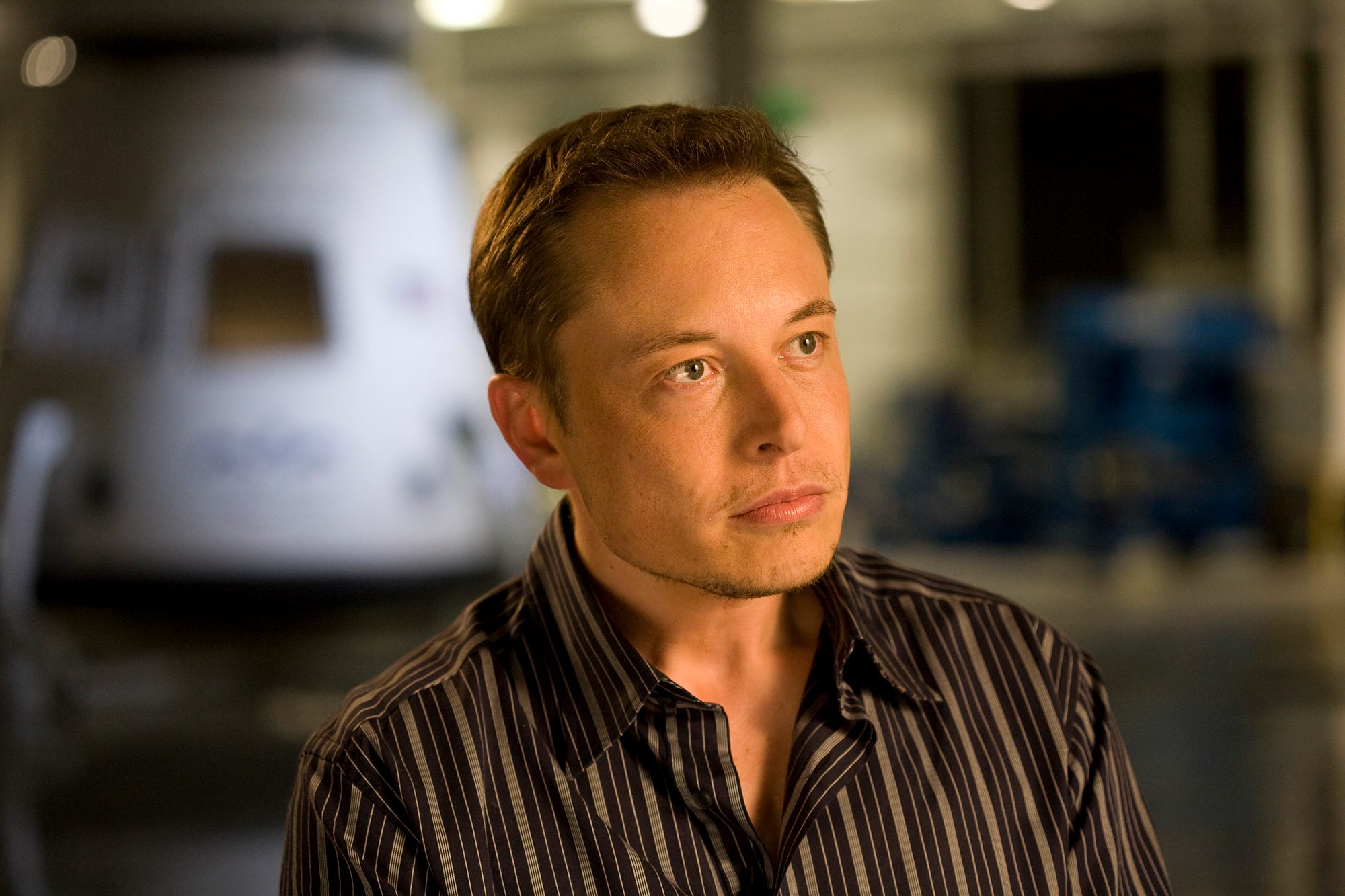
Word leaked Monday via The Wall Street Journal that Tesla / SpaceX industrialist Elon Musk has been funding a company called Neuralink— allegedly with some of his own money — attempting to connect computers directly into human brains. This is the same Musk profiled in this month’s Vanity Fair, where he tells journalist Maureen Dowd in all seriousness that humanity needs a Mars colony to which we can escape ‘if AI goes rogue and turns on humanity.”
Which side is he on?
In short, Musk is one of many big thinkers who believe a human-computer hybrid is essential to allowing humans to keep their own machines from marginalizing them. Neuralink’s technology is said to be a neural lace, which Musk has spoken about for over a year.
But for most people, the first question isn’t whether artificial intelligence will usurp our planet. The first question is: What exactly is AI?
Let’s skip the science fiction and get to the science: AI research and development spans a broad range of fields and myriad goals, as befits the concept of mimicking the vast breadth and depth of a human mind as compared to a calculator. Even people who work in AI, and reporters who’ve covered it for years, can’t agree on what doesn’t and doesn’t count as “intelligence,” or how to group all AI projects into a few understandable categories. We tried asking.
Five Types of AI
The explanation-friendly people at Tutorials Point have done a tidy job of breaking AI research into an understandable graphic with five major categories. (Their tutorial is a good next step to learn more details about AI research areas.)
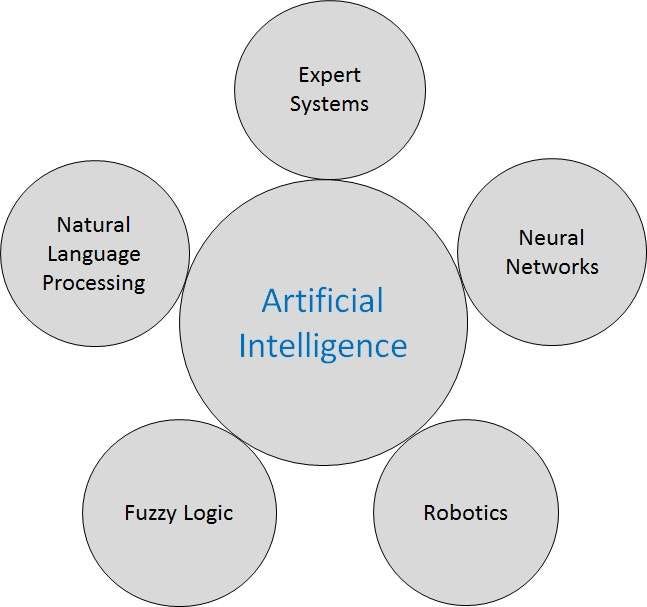
Expert Systems
These are computer systems which are programmed with vast histories of human expertise on a topic, so that they can quickly examine far more options, scenarios and solutions than a team of experts could ever come up with. Google Maps, which solves the apocryphal traveling salesman’s dilemma before you’ve realized you have one, is a familiar example. Air traffic control systems juggle even bigger arrays of data points, options and restrictions.
Clinical systems, which dispense medical advice, are one of the promising areas for AI — what doctor can keep on top of all medical knowledge today, even in one field? But doctors point out that these systems are a long way from replacing a human clinician — they’re helpful advisers, not successors.
Neural Networks
Artificial neural network systems somewhat mimic the neurons in the human brain. They are already far superior to humans at tasks that involve pattern-matching. They can spot a face in a crowd from old photos, or tell you not only what someone said or wrote, but who like said it or wrote it (or didn’t!) based on language patterns too subtle and complex for mere mortals to spot.
Fuzzy Logic
Traditional computer programs work with hard logic — true or false, 1 or 0, yes or no. Fuzzy logic allows an array of possible values, a sliding scale of trueness, a “truthiness” rather than the inflexible numbers of a spreadsheet. It’s much more like how humans think.
Your washing machine may already use fuzzy logic — that’s how it can do one-touch cleaning. But more advanced fuzzy logic is what will enable a self-driving car. If it’s going to run over one baby or five old people, which will it choose? Silly human, you’re looking for there to be one right answer.
Robotics
Most babies learn to walk in less than a year. Many animals can scamper up, over, and around terrain where humans wouldn’t dare. Researchers are learning a simple truth: Walking is hard. Getting a robot to take a single step under its own balance took years. That second step is a doozy.
But robotic systems are already much better than people at many precision tasks, such as assembling products or shipping boxes from an Amazon warehouse. Not only are they precise, they’re smart — they scan spot production glitches or incorrect parts, and can compensate for variances from one product to the next.
Even products that require craftsmanship, like building guitars, can often be done better, faster and more reliably by CNC manufacturing now. It’s getting harder for experienced players to tell a $400 robot-made G&L guitar from Indonesia — or is it China now? — from a $1,500 one built by hand in California.
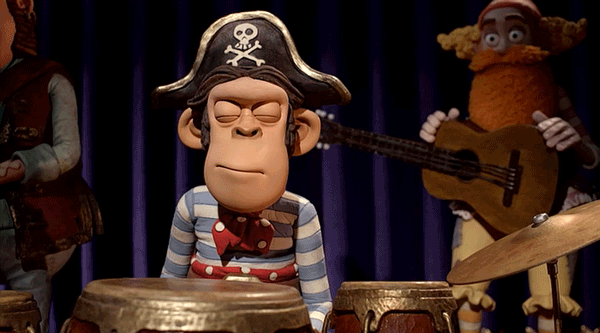
AI for Chatbots: Natural Language Processing
NLP, as everyone calls it, is the corner of AI most applicable to chatbots. The goal of natural language processing is to hold everyday conversations with humans, without them needing to speak in restricted syntaxes and vocabularies, or speak (or type) special commands. Researcher Alan Turing proposed a simple test for a successful program: “A computer would deserve to be called intelligent if it could deceive a human into believing that it was human.” By that standard, the Twitterbots have already won.
But as Pandorabots founder Lauren Kunze, who built her first chatbot at age fifteen, told us recently, “Walking is complex, language is far beyond that.” Human languages are far more complex than computer programming languages in complexity, flexibility, malleability and nuance. As a human, you can read a 500-year-old play by Shakespeare and sort of tell what’s going on. Try typing some Shakespeare at an Internet chatbot.
Musk told Vanity Fair that he believes a human-brain interface is four to five years away. But IBM researchers once claimed they would have software that could not only understand any human language, but translate it into any other language, in three years. That was in 1954.
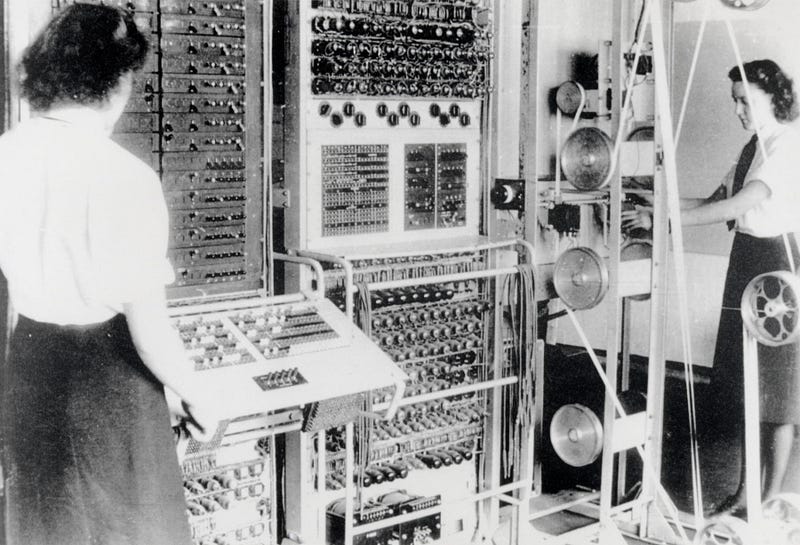
A Never-Ending Quest
Artificial intelligence — hardware and software that performs functions once believed possible only by a living brain — has been a concrete goal of technologists for more than 150 years, since Charles Babbage drafted the design for his Difference Engine (never built until the 1990’s, as a museum piece) and Ada Lovelace realized it could manipulate not just numbers, but musical notes or anything else with a formulaic system.
But the path from vision to reality is one that continues to get longer and longer the further we travel down it. Dead ends, imminent breakthroughs that never happen, and algorithms that almost work have become pretty much expected of any direction AI research takes.
The people who pay for that research frequently become disillusioned. Scientists talk about the AI Winters of the 1970’s and 1980’s, when one institution after another refused to pour more money into projects that were neither sticking to schedule nor delivering the hoped-for results.
AI took a mini-hit this past year in the world of chatbots. Facebook’s announcement of an AI-enabling platform for its Messenger communication channel spurred a slew of investments in automated friends, assistants and services (including Octane AI, which publishes Chatbots Magazine.) A year later, reports claimed that Facebook’s M project, advanced AI designed to understand Messenger users, could comprehend and complete fewer than one in three requests.
Moreover, AI research today is unlike the Internet R&D that pawns thousands of products from a never-ending stream of small startup companies. Modern AI research uses an almost unimaginable amount of computing power and time — beyond the financial reach of many startups. And engineers who understand sub-disciplines like machine learning — whereby one doesn’t program software directly, but instead gives it an ocean of example data from which to learn on its own — are rare and therefore expensive, even for computer programmers.
That’s why startup investment firm Y Combinator recently announced a special funding track for AI startups. Y Combinator plans to provide entrepreneurs whose ideas seem financially promising with extra computational credits for cloud computing, and with experts in machine learning consultants who will make office hours to help young founders. Those are what Google, Facebook, IBM and other big-budget firms can afford that two Stanford dropouts in a loft can’t.
So What Are Musk and Others Afraid Of?
Many discussions of artificial intelligence skip past what it is or isn’t to an apocalyptic worry: That a sufficiently complex computer system will develop self-awareness, literally thinking for itself. And that one or more such artificial superminds will then decide the pesky humans who built them are in the way. The Vanity Fair article is a well-written primer on who worries about what among leading tech thinkers.
But one reason world-conquering AI seems to always be just a few more years out is that to us humans, advanced software only earns the title of “artificial intelligence” until it becomes part of everyday life. What we once imagined only a human mind could deduce, like finding the fastest driving route through five spots across Los Angeles, loses its mystique once Lyft does it. Dude, it’s just an app.
It’s been deemed the AI effect, tidily summarized as Tesler’s Law: “AI is whatever hasn’t been done yet.”
This series continues Wednesday with “What is NLP?” and Thursday with “What is Machine Learning?
Click the ❤ below to recommend this story to other Medium readers looking to learn more about the world of AI and chatbots.
from Chatbots Magazine – Medium https://chatbotsmagazine.com/what-is-ai-even-elon-musk-cant-explain-1070b492d3d5?source=rss—-d6dc2c824f17—4